In this post, I install the new DBeaver OpenAI ChatGPT integration, try it out with some prompts and examine my OpenAI usage costs.
Table of Contents
Introduction
DBeaver is a free database tool and SQL client. It is multi-platform, open-source and supports a variety of databases including Microsoft SQL Server, Amazon Athena and MySQL.
In February 2023, the latest version of DBeaver’s Community Edition was released. Version 22.3.4’s release notes start with:
ChatGPT integration for smart completion and code generation (as optional extension).
ChatGPT has been in the news a lot since its launch in November 2022. It has earned widespread praise and scorn, has been embraced in some places and banned in others and has attracted billions of dollars of investment.
So how does ChatGPT fare in this setting? Let’s find out!
Preparation
In this section, I install and configure DBeaver’s OpenAI ChatGPT integration.
DBeaver’s OpenAI ChatGPT integration is not automatically installed with Version 22.3.4. This makes sense – it’s currently quite new and many users and organisations may not want to install it (for reasons I’ll go into later). This approach keeps DBeaver’s footprint as small as possible and lets people make up their own minds.
DBeaver’s GitHub wiki covers the installation process, which amounts to:
- Open DBeaver’s integration installer.
- Select the
DBeaver AI (GPT) integration
library. - Select and install
DBeaver AI (GPT) Support
.
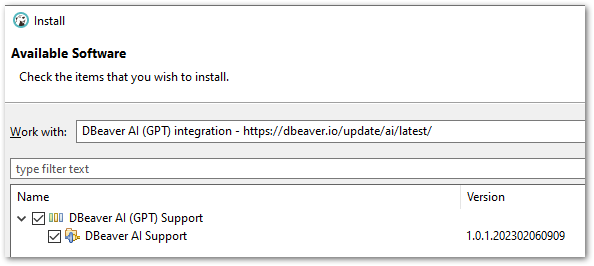
Following this, the DBeaver OpenAI ChatGPT integration must be configured. This process needs an active API token from the OpenAI platform.
OpenAPI account holders can generate tokens in the API Keys section of their profile. Afterwards, this token must be pasted into the API Token
field in DBeaver’s preferences:
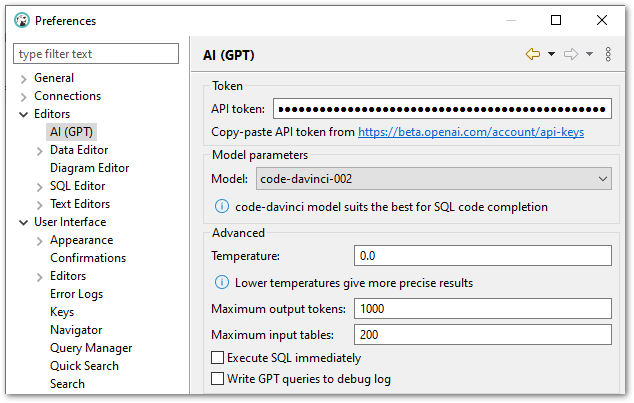
The DBeaver OpenAI ChatGPT integration will now work. The other settings are out of scope for this post and are covered in DBeaver’s wiki.
Separately, I found I was getting SQL 08S01
timeout errors during my first attempts to send ChatGPT prompts. I ended up increasing my database connection’s connectionTimeout
value to resolve this.
ChatGPT Data Sharing
There is an important fact to consider when using DBeaver’s OpenAI ChatGPT integration:
DBeaver needs to send database metadata to the OpenAI platform.
To clarify, this means that DBeaver sends a list of the selected database’s table names and column names to OpenAI.
DBeaver doesn’t hide this fact away. Their wiki is very explicit, and the DBeaver OpenAI ChatGPT integration states this when used for the first time.
For my purposes, this isn’t a problem. My amazonwebshark MySQL database contains nothing confidential or sensitive, and the tables and columns of a standard WordPress database are well documented.
But I wouldn’t currently use this with databases I don’t own. Although the shared metadata is limited to table names and column names, these can still be sensitive and potentially misused. I’m sure OpenAI have data security measures in place, but as this technology is still quite new I currently prefer to be cautious.
Tests
In this section, I test the DBeaver OpenAI ChatGPT integration with prompts referring to my amazonwebshark MySQL database. Before starting, I should define the concepts of prompts and completions:
- Prompts are the instructions given to ChatGPT.
- Completions are ChatGPT’s response to prompts.
Test 1: Category Usage
For my first test, I wanted to answer a question similar to one from my last post:
How many amazonwebshark blog categories have been used more than twice?
I answered the question with this SQL query:
SELECT t.name, tt.count
FROM term_taxonomy AS tt
INNER JOIN terms AS t
ON tt.term_id = t.term_id
WHERE tt.taxonomy = 'category'
AND tt.count > 2
Which returned these results:
name | count |
Data & Analytics | 6 |
Me | 4 |
Developing & Application Integration | 8 |
Security & Monitoring | 3 |
Now it’s ChatGPT’s turn! My first prompt for ChatGPT was:
Find all categories used more than 2 times.
GPT’s completion was:
SELECT COUNT(*) AS count, category
FROM statistics_historical
GROUP BY category
HAVING count > 2;
Although this query looks fine, it doesn’t return any results! In fact the whole table has nothing in it:
SELECT COUNT(*) FROM statistics_historical
Name | Value |
COUNT(*) | 0 |
ChatGPT interpreted my prompt literally and searched my database metadata for tables with a category
column. This search only returned one table, which is used for archiving purged report data: statistics_historical
.
ChatGPT didn’t know that the table was empty, and so had no way to know its query wasn’t appropriate. This was my fault – my prompt needed more work.
Test 2: Category Usage Take 2
For my second test, I tried to re-engineer my prompt to give ChatGPT more insight into my data. My question remained:
“How many amazonwebshark blog categories have been used more than twice?”
My second prompt was phrased to give ChatGPT some guidance on what to look for:
Get all names of terms with taxonomy of category and count greater than 2
This time, GPT’s completion was:
SELECT name, count
FROM terms
INNER JOIN term_taxonomy
ON terms.term_id = term_taxonomy.term_id
WHERE term_taxonomy.taxonomy = 'category'
AND term_taxonomy.count > 2
This was almost identical to my own SQL query:
SELECT t.name, tt.count
FROM term_taxonomy AS tt
INNER JOIN terms AS t
ON tt.term_id = t.term_id
WHERE tt.taxonomy = 'category'
AND tt.count > 2
And the results were the same in both cases:
name | count |
Data & Analytics | 6 |
Me | 4 |
Developing & Application Integration | 8 |
Security & Monitoring | 3 |
Test 3: Page Views
For my final test I tried a different approach. Instead of writing a SQL query myself, I gave ChatGPT a prompt and then used a WPStatistics report to compare results.
My question is now:
Which amazonwebshark posts have had the highest views in the past week?
I know which table has the data needed for this, so I included it along with some vital columns in my ChatGPT prompt:
Get the uris for the past week from statistics_pages. Sum all the counts. Show the top 5 in descending order.
GPT’s completion was:
SELECT uri, SUM(count) AS total_count
FROM statistics_pages
WHERE date >= DATE_SUB(CURDATE(), INTERVAL 7 DAY)
GROUP BY uri
ORDER BY total_count DESC
LIMIT 5;
This was impressive! ChatGPT used the table and columns I specified, filtered the dates correctly and limited the results as instructed.
The query results were:
uri | total count |
/connecting-athena-to-power-bi-with-simba-athena/ | 31 |
/writing-user-stories-for-an-itunes-dashboard/ | 24 |
/using-athena-to-query-s3-inventory-parquet-objects/ | 22 |
/s3-glacier-instant-retrieval-first-impressions/ | 10 |
/open-source-documentation-with-read-the-docs/ | 7 |
I compared these with my WPStatistics Pages report:
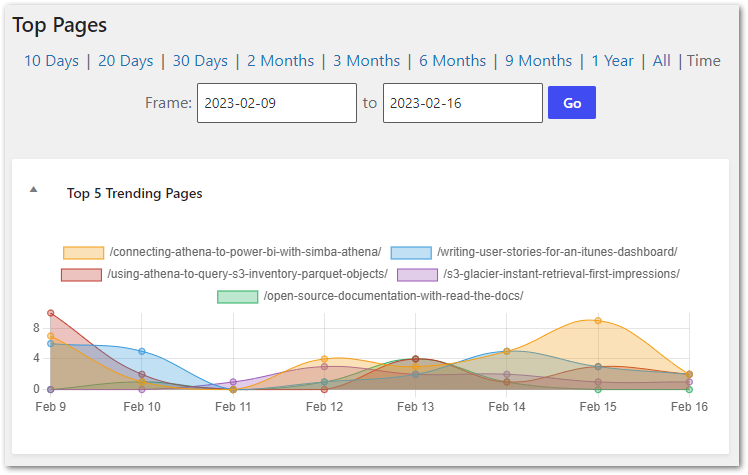
And the results were the same!
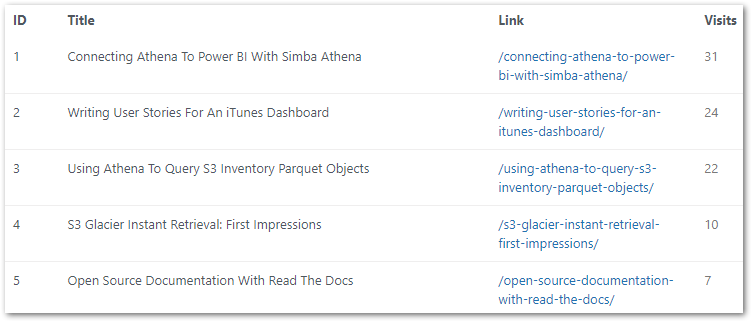
Pricing
In this section, I examine OpenAI’s usage and billing services.
Tokens
OpenAI uses the concept of tokens to calculate pricing. In their words:
The GPT family of models process text using tokens, which are common sequences of characters found in text. The models understand the statistical relationships between these tokens, and excel at producing the next token in a sequence of tokens.
OpenAI Tokenizer site
And:
“You can think of tokens as pieces of words used for natural language processing. For English text, 1 token is approximately 4 characters or 0.75 words. As a point of reference, the collected works of Shakespeare are about 900,000 words or 1.2M tokens.”
OpenAI Pricing site
OpenAI currently offers several tools for determining pricing:
- Pricing site for costs in USD.
- Tokenizer site for showing how the API will tokenize text.
- transformers package for Python.
- gpt-3-encoder package for node.js.
OpenAI also operates a free tier. New users get $18 worth of free tokens. These expire after 3 months, after which accounts can be upgraded to paid plans operating on a pay-as-you-go basis. At this point, accounts with no billing information can still be accessed but no further API requests will be granted.
Usage
Here is my OpenAI usage for 2023-02-15:
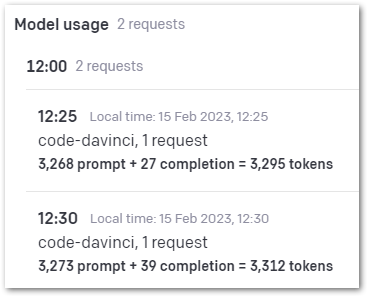
And 2023-02-16:
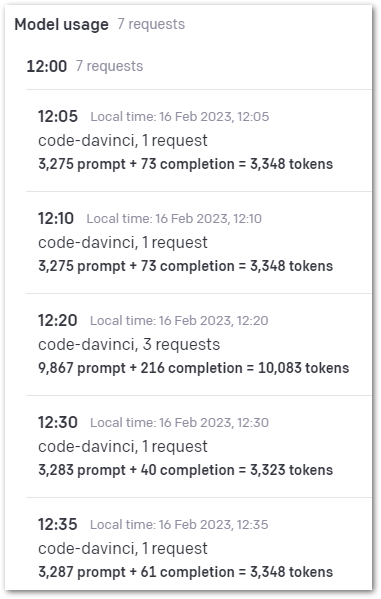
To understand this better, let’s examine the last row that relates to Test 3. The values stated are:
- 3284 prompt tokens.
- 61 completion tokens.
The Completion value is easy to verify. Entering ChatGPT’s Test 3 completion into the tokenizer site scores it at 61 tokens:
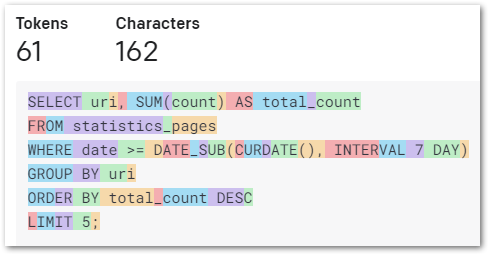
Now let’s examine the Prompt value. When I enter my Test 3 prompt into the tokenizer site, it only scores it at 25 tokens. I wanted to see where the other 3259 tokens came from, so I needed to see what DBeaver sent to ChatGPT.
Currently, my OpenAI account doesn’t keep a record of what their APIs send and receive. However, I can log what DBeaver sends to OpenAI! This is done by enabling the Write GPT queries to debug log
option in DBeaver’s ChatGPT settings.
It turns out that DBeaver includes DDL statements for all of the tables and columns in my amazonwebshark MySQL database in every ChatGPT prompt. Even in cases like Test 3 where I tell ChatGPT which table to use!
This isn’t unreasonable, as ChatGPT’s information retention is limited and it can’t access past conversations to inform its responses. But this explains the high prompt values per request. The OpenAI tokeniser says Test 3’s full prompt is 8293 characters. And remember – every 4 characters requires 1 token!
Addendum: DBeaver version 22.3.5 added metadata scope configuration to their ChatGPT smart completion.
In terms of size, my amazonwebshark MySQL database has around 20 tables with modest column counts. Larger databases with dozens of tables and potentially hundreds of columns could quickly rack up high usage fees, so use caution in these situations.
Billing
OpenAI has features to help with billing. Their site stores payment methods and shows invoices from the past 12 months. In addition, preferences like additional email addresses for billing notifications and business tax ID retention can be specified.
Accounts can also have usage limits, triggering notification emails when reached. These work alongside the usage tracking dashboard to help monitor spending.
So how much have I spent here?
By default, DBeaver uses the DaVinci model. This is currently listed at $0.0200 per 1000 tokens. If I take the 30,057 total tokens I’ve used and price them at $0.0200 per 1000 tokens, I get $0.60114.
In GBP that’s about £0.50 (or about 5p per query) which my free tier will cover.
Summary
In this post, I installed the new DBeaver OpenAI ChatGPT integration, tried it out with some prompts and examined the OpenAI usage costs.
Overall I was very impressed with what I found! ChatGPT works really well with a well-engineered prompt. It understood what I wanted and worked quickly. Would I pay for it? I think so, provided I kept an eye on the prompts being sent and the resulting usage costs.
In a future version, I’d like to be able to control what database metadata is included in a prompt. This would let me make sure that ChatGPT wasn’t getting sensitive or unnecessary metadata, and the smaller prompts would reduce my API usage costs.
Addendum: DBeaver version 22.3.5 added metadata scope configuration to their ChatGPT smart completion.
This is all very exciting though! I’m interested to discover how else the DBeaver OpenAI ChatGPT integration can help me out, and fascinated to see future developments!
If this post has been useful, please feel free to follow me on the following platforms for future updates:
Thanks for reading ~~^~~