In this post, I try Tobias Müller‘s free SQL Workbench tool powered by DuckDB-Wasm. And maybe make one or two gratuitous duck puns.
Table of Contents
Introduction
Hopefully like many people in tech, I have a collection of articles, repos, projects and whatnot saved under the banner of “That sounds cool / looks interesting / feels useful – I should check that out at some point.” Sometimes things even come off that list…
DuckDB was on my list, and went to the top when I heard about Tobias Müller’s free SQL Workbench tool powered by DuckDB-Wasm. What I heard was very impressive and pushed me to finally examine DuckDB up close.
So what is DuckDB?
About DuckDB
This section examines DuckDB and DuckDB-Wasm – core components of SQL Workbench.
DuckDB
DuckDB is an open-source SQL Online Analytical Processing (OLAP) database management system. It is intended for analytical workloads, and common use cases include in-process analytics, exploration of large datasets and machine learning model prototyping. DuckDB was released in 2019 and reached v1.0.0 on June 03 2024.
DuckDB is an embedded database that runs within a host process. It is lightweight, portable and requires no separate server. It can be embedded into applications, scripts, and notebooks without bespoke infrastructure.
DuckDB uses a columnar storage format and supports standard SQL features like complex queries, joins, aggregations and window functions. It can be used with programming languages like Python and R.
In this Python example, DuckDB:
- Creates an in-memory database
- Defines a
users
table. - Inserts data into
users
. - Runs a query to fetch results.
import duckdb
# Create a new DuckDB database in memory
con = duckdb.connect(database=':memory:')
# Create a table and insert some data
con.execute("""
CREATE TABLE users (
user_id INTEGER,
user_name VARCHAR,
age INTEGER
);
""")
con.execute("INSERT INTO users VALUES (1, 'Alice', 30), (2, 'Bob', 25), (3, 'Charlie', 35)")
# Run a query
results = con.execute("SELECT * FROM users WHERE age > 25").fetchall()
print(results)
DuckDB-Wasm
Launched in 2021, DuckDB WebAssembly (Wasm) is a version of the DuckDB database that has been compiled to run in WebAssembly. This lets DuckDB run in web browser processes and other environments with WebAssembly support.
So what’s WebAssembly? Don’t worry – I didn’t know either and so deferred to an expert:
Since all data processing happens in the browser, DuckDB-Wasm brings new benefits to DuckDB. In-browser dashboards and data analysis tools with DuckDB-Wasm enabled can operate without any server-side processing, improving their speed and security. If data is being sent somewhere then DuckDB-Wasm can handle ETL operations first, reducing both processing time and cost.
Running in-browser SQL queries also removes the need for setting up database servers and IDEs, reducing patching and maintenance (but don’t forget about the browser!) while increasing availability and convenience for remote work and educational purposes.
Like DuckDB, it is open-source and the code is on GitHub. A DuckDB Web Shell is available at shell.duckdb.org and there are also more technical details on DuckDB’s blog.
SQL Workbench
This section examines SQL Workbench and tries out some of its core features.
About SQL Workbench
Tobias Müller produced SQL Workbench in January 2024. He integrated DuckDB-Wasm into an AWS serverless static site and built an online interactive tool for querying data, showing results and generating visualizations.
SQL Workbench is available at sql-workbench.com. Note that it doesn’t currently support mobile browsers. Tobias has also written a great tutorial post that includes:
and loads more content in addition that I won’t reproduce here. So go and give Tobias some traffic!
Layout
The core SQL Workbench components are:
- The Object Explorer section shows databases, schema and tables:
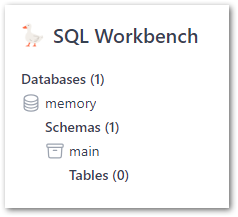
- The Tools section shows links, settings and a drag-and-drop section for adding files (more on that later):
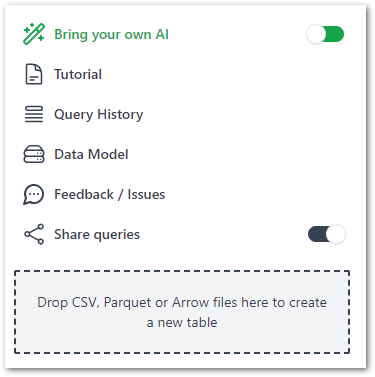
- The Query Pane for writing SQL, which preloads with the below script upon each browser refresh:
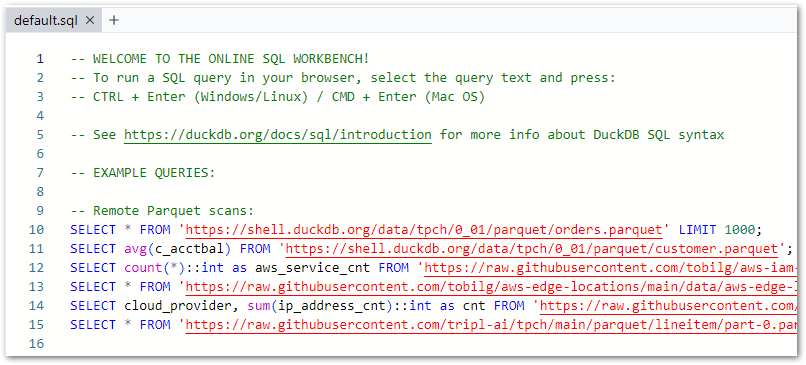
- And finally, the Results Pane shows query results and visuals:
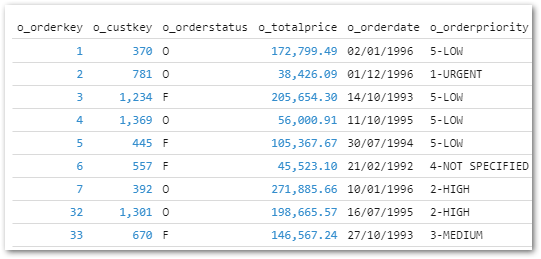
Next, let’s try it out!
Sample Queries
SQL Workbench opens with a pre-loaded script that features:
- Instructions:
-- WELCOME TO THE ONLINE SQL WORKBENCH!
-- To run a SQL query in your browser, select the query text and press:
-- CTRL + Enter (Windows/Linux) / CMD + Enter (Mac OS)
-- See https://duckdb.org/docs/sql/introduction for more info about DuckDB SQL syntax
- Example queries using Parquet files:
-- Remote Parquet scans:
SELECT * FROM 'https://shell.duckdb.org/data/tpch/0_01/parquet/orders.parquet' LIMIT 1000;
SELECT avg(c_acctbal) FROM 'https://shell.duckdb.org/data/tpch/0_01/parquet/customer.parquet';
SELECT count(*)::int as aws_service_cnt FROM 'https://raw.githubusercontent.com/tobilg/aws-iam-data/main/data/parquet/aws_services.parquet';
SELECT * FROM 'https://raw.githubusercontent.com/tobilg/aws-edge-locations/main/data/aws-edge-locations.parquet';
SELECT cloud_provider, sum(ip_address_cnt)::int as cnt FROM 'https://raw.githubusercontent.com/tobilg/public-cloud-provider-ip-ranges/main/data/providers/all.parquet' GROUP BY cloud_provider;
SELECT * FROM 'https://raw.githubusercontent.com/tripl-ai/tpch/main/parquet/lineitem/part-0.parquet';
- Example query using a CSV file:
-- Remote CSV scan
SELECT * FROM read_csv_auto('https://raw.githubusercontent.com/tobilg/public-cloud-provider-ip-ranges/main/data/providers/all.csv');
These queries are designed to show SQL Workbench’s capabilities and the style of SQL queries it can run (expanded on further here). They can also be used for experimentation, so here goes!
Firstly, I’ve added an ORDER BY
to the first Parquet query to make sure the first 1000 orders are returned:
-- Show first 1000 orders for Parquet file
SELECT *
FROM 'https://shell.duckdb.org/data/tpch/0_01/parquet/orders.parquet'
ORDER BY o_orderkey
LIMIT 1000;
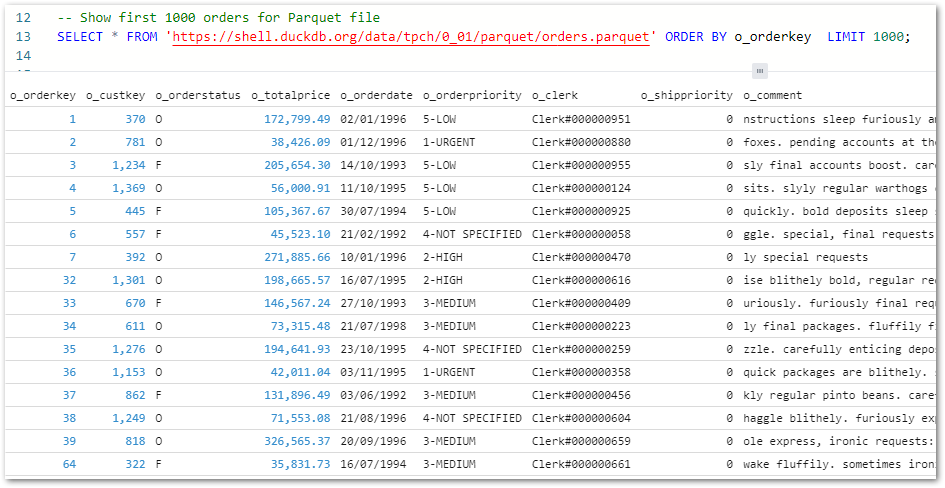
Secondly, I’ve used the COUNT
and CAST
functions to count the total orders and return the value as an integer:
-- Show number of orders for Parquet file
SELECT CAST(COUNT(o_orderkey) AS INT) AS orderkeycount
FROM 'https://shell.duckdb.org/data/tpch/0_01/parquet/orders.parquet';
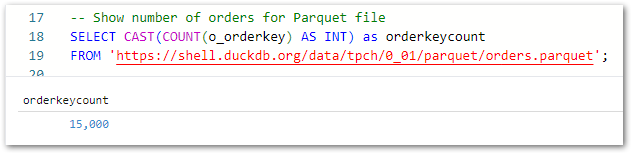
Finally, this query captures all 1996 orders in a CTE and uses it to calculate the 1996 order price totals grouped by order priority:
-- Show 1996 order totals in priority order
WITH cte_orders1996 AS (
SELECT o_orderkey, o_custkey, o_totalprice, o_orderdate, o_orderpriority
FROM 'https://shell.duckdb.org/data/tpch/0_01/parquet/orders.parquet'
WHERE YEAR(o_orderdate) = 1996
)
SELECT SUM(o_totalprice) AS totalpriorityprice, o_orderpriority
FROM cte_orders1996
GROUP BY o_orderpriority
ORDER BY o_orderpriority;
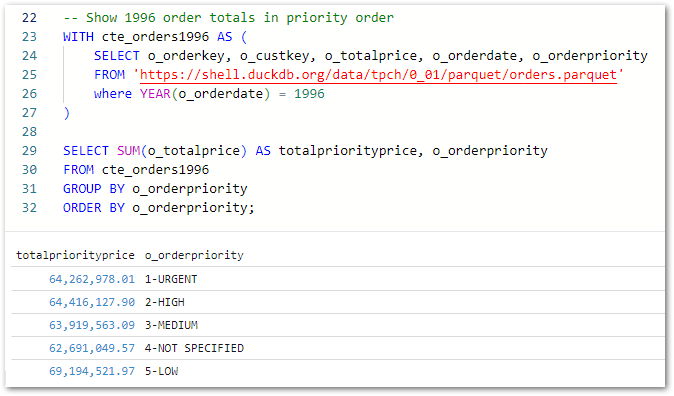
Now let’s try some of my data!
File Imports
SQL Workbench can also query data stored locally (as well as remote data which is out of this post’s scope). For this section I’ll be using some Parquet files generated by my WordPress bronze data orchestration process: posts.parquet
and statistics_pages.parquet
.
After dragging each file onto the assigned section they quickly appear as new tables:
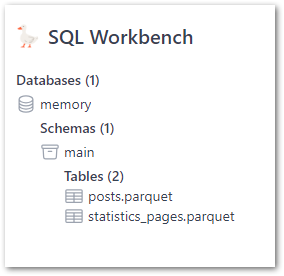
These tables show column names and inferred data types when expanded:
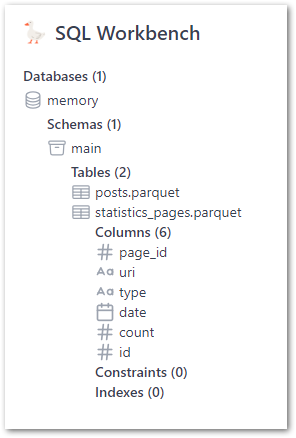
Queries can be written manually or by right-clicking the tables in the Object Explorer. A statistics_pages
SELECT *
test query shows the expected results:
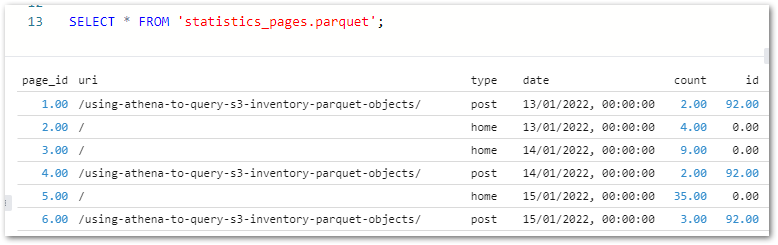
As posts.parquet
‘s ID primary key column matches statistics_pages.parquet
‘s ID foreign key column, the two files can be joined on this column using an INNER JOIN
:
SELECT
p.post_title,
sp.type,
sp.date as viewdate,
sp.count
FROM
'statistics_pages.parquet' AS sp
INNER JOIN 'posts.parquet' AS p ON sp.id = p.id
ORDER BY sp.date
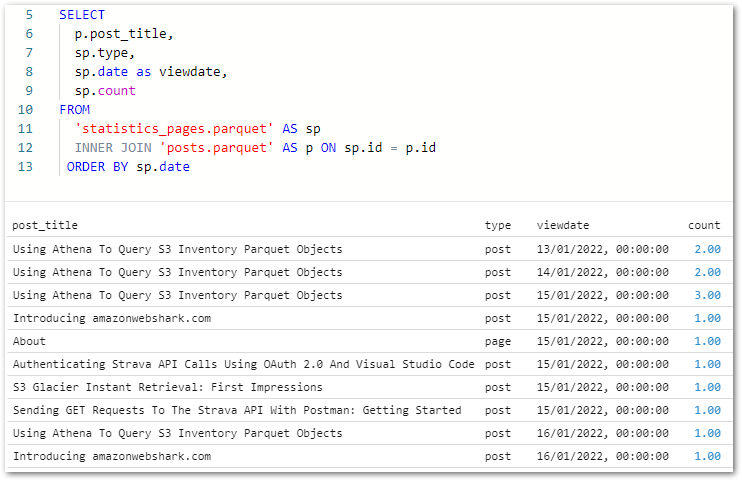
I can use this query to start making visuals!
Visuals
Next, let’s examine SQL Workbench’s visualisation capabilities. To help things along, I’ve written a new query showing the cumulative view count for my Using Athena To Query S3 Inventory Parquet Objects post (ID 92):
SELECT
sp.date AS viewdate,
SUM(sp.count) OVER (PARTITION BY sp.id ORDER BY sp.date) AS cumulative_views
FROM
'statistics_pages.parquet' AS sp
INNER JOIN 'posts.parquet' AS p ON sp.id = p.id
WHERE p.id = 92
ORDER BY sp.date;
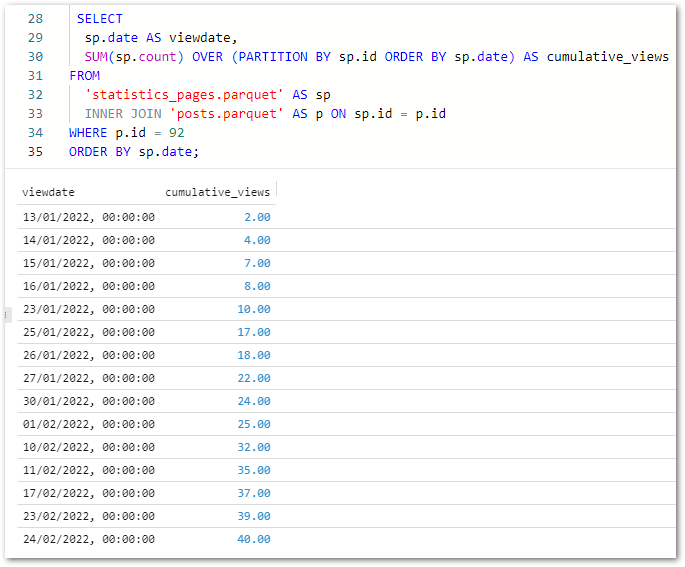
(On reflection the join wasn’t necessary for this output, but anyway… – Ed)
Tobias has written visualisation instructions here so I’ll focus more on how my visual turns out. After the query finishes running, several visual types can be selected:
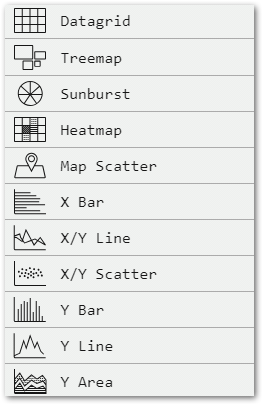
Selecting a visual quickly renders it in the browser. Customisation options are also available. That said, in my case the first chart was mostly fine!
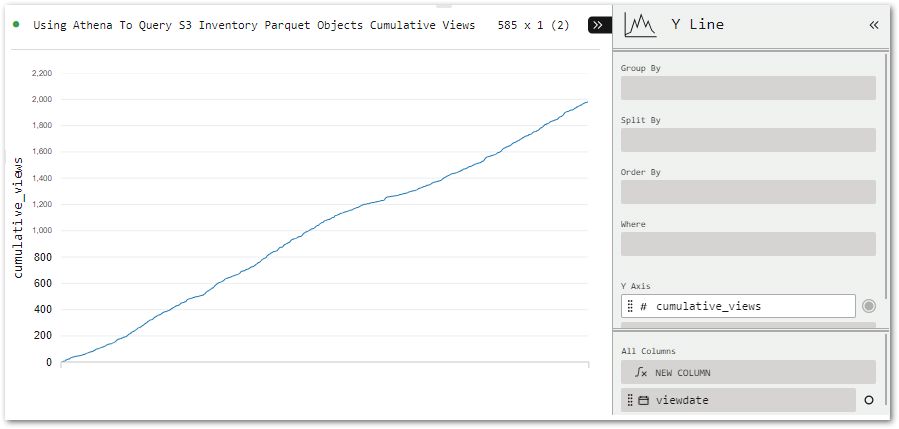
All I need to do is move viewdata
(bottom right) to the Group By bin (top right) to add dates to the X-axis.
Exports & Cleanup
Finally, let’s examine SQL Workbench’s export options. And there’s plenty to choose from!
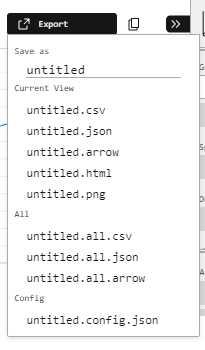
The CSV
and JSON
options export the query results in their respective formats. The Arrow
option exports query results in Apache Arrow format, which is designed for high-speed in-memory data processing and so compliments DuckDB very well. Dremio wrote a great Arrow technical guide for those curious.
The HTML
option produces an interactive graph with tooltips and configuration options. Handy for sharing and I’m sure there’ll be a way to use the backend code for embedding if I take a closer look. The PNG
has me covered in the meantime, immediately producing this image:
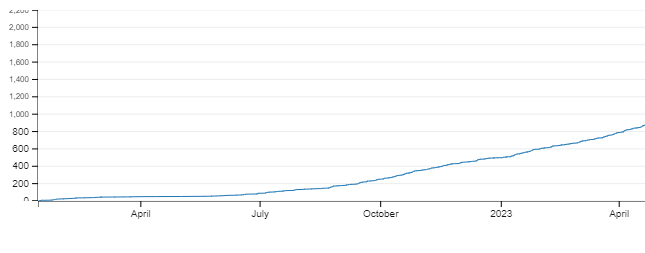
Finally there’s the Config.JSON
option, which exports the visual’s settings for version control and reproducibility:
{"version":"2.10.0","plugin":"Y Line","plugin_config":{},"columns_config":{},"settings":true,"theme":"Pro Light","title":"Using Athena To Query S3 Inventory Parquet Objects Cumulative Views","group_by":["viewdate"],"split_by":[],"columns":["cumulative_views"],"filter":[],"sort":[],"expressions":{},"aggregates":{}}
All done! So how do I remove my data from SQL Workbench? I…refresh the site! Because SQL Workbench uses DuckDB, my data has only ever been stored locally and in memory. This means the data only persists until the SQL Workbench page is closed or reloaded.
Summary
In this post, I tried Tobias Müller’s free SQL Workbench tool powered by DuckDB-Wasm.
SQL Workbench and DuckDB are both very impressive! The results they produce with no servers or data movement are remarkable, and their tooling greatly simplifies working with modern data formats.
DuckDB makes lots of sense as an AWS Lambda layer, and DuckDB-Wasm simplifies in-browser analytics at a time when being data-driven and latency-averse are constantly gaining importance. I don’t feel like I’ve even scratched the surface of SQL Workbench either, so go and check it out!
Phew – no duck puns. No one has time for that quack. If this post has been useful then the button below has links for contact, socials, projects and sessions:
Thanks for reading ~~^~~